The past two years – while I was working as a consultant on an AI project in the banking sector – have given me some valuable insights into the current developments in the field of data and analytics and how we are able to generate business value with those tools. It is beyond a reasonable doubt that AI has a huge impact on the banking industry (and all other industries as well). But how can you unleash the potential of AI exactly? My lessons learnt are focusing on these 3 areas: data, process and team set up.
Opening a bank’s data vault
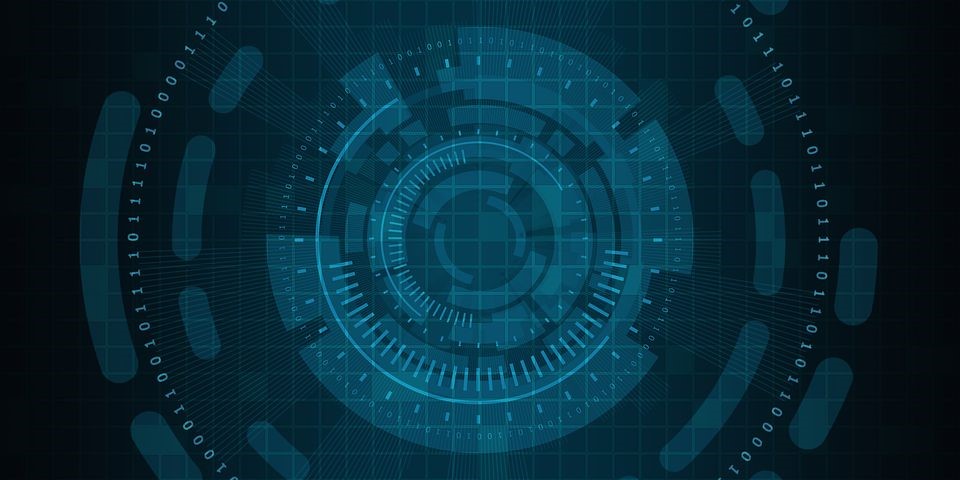
Don’t start a project without data or as 50 Cent would say: “Get data or die tryin”. This might sound very trivial to you but unfortunately the reality looks different.
But here comes the good news: banks are sitting on a data gold mine. There is already a huge amount of data stored (somewhere). Combining data in new ways and evolve algorithms make it possible to unlock new trends and growth (e.g. cross-selling).
But what really caught my eye was the fact that many people in big corporations are still not aware that a machine learning algorithm is only as good as the data it’s fed. So, if someone comes to you with a data set of 10 entries and expects that some algorithm will be able to learn enough insights to be applicable to thousands of real world problems, forget it (for now)…AI is not a magic wand! However, there are already some sort of libraries that can be trained to learn domain knowledge with a small set of documents, because they rely on a more generic model (see also https://sifted.eu/articles/ai-fintech-eigen/).
As I am already bringing up the subject of model training, I am inclined to also mention that most use cases in the field of ML are still based on supervised learning which requires labelled data. So be prepared to solve annotation issues first before thinking about building up a ML model. Some might use manpower by potentially offshoring the manual labelling effort to a low-cost country or buy an expensive annotation tool. BUT before taking any decisions, please check first if there is already some information available in any systems. A concrete example: Even though we had enough client documents to train the model, we had to label documents manually onshore (since we are not allowed to use client information data outside of Switzerland). We started with 20-30 documents but by chance we discovered that this data had already been manually typed into one of the many databases in the complex banking storage system. Thus, we could easily generate automatic labelling with it.
All in all, it is vital for a successful AI project to have enough data, a good data understanding and preparation before even starting with training the model with available data.
„Fail early, fail often but always fail forward“ – John C. Maxwell
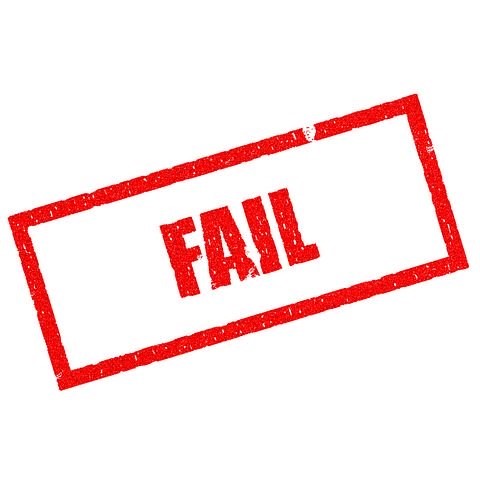
While establishing an AI project / platform / center of excellence within a company, I learned it is crucial to not start the discussion with how to establish a data lake or how to build an AI platform. Begin with a POC (proof of concept) before thinking of something else and this is the best piece of advice I can give. I know that many clients want a RFP and a full-stretched cost estimation upfront, but this is just not possible! Why? Especially in the field of AI/ML, the process works best in cycles and you never know in advance if something would work. By means of doing this you can evaluate after each cycle if the ML model works, easily detect issues that you might not have thought of (e.g. legal issues) and be able to better estimate the total effort for the end product. One learning out of this could also be that the chosen approach was wrong and there is no silver lining! So if it fails, then at least you have not invested too much time and money already in the product. On top of that, what I have experienced is also that the client prefers this new way of pitching with a small demo of the POC. Especially business stakeholders and project sponsors can then better understand the product and assess the potential benefits and risks. The wow effect is guaranteed!
Another key action that should be done at the latest during the POC phase is to make sure you understand the end-to-end business process and also be able to challenge existing flows. Building up a ML model for documents that is only used for 100 clients per year might not create enough business value and ROI. Having fancy AI in the process is fair enough but it is important to ask whether the project is really in need of AI.
To summarize, POCs are a suitable approach in the field of AI. They allow fast model validation and testing of use cases. BUT bear in mind, POC is not the final stage. At some point, you need to generate value with the product and bring it into production. By using these POC use cases, you can successfully build up the AI platform in an iterative process.
Keep AI team away from production support
We started the AI journey two and a half years ago. Until now we have brought some of our initial POCs into life (deployed into production). But what has not changed yet is the fact that we are still operating as ONE team. Meaning, that we split our work between handling new requests, generating POCs, and also maintaining our solution in production. Oftentimes, if a production issue arises while the team is working on new POCs, the clients always (and of course) prioritize to fix the production issue first. This leads to inefficiency due to task switch and demotivation of the team. Here (and this is just my two cents), AI projects could be improved by splitting the team into (at least) two teams. One team would function as a decentralized “POC” team which consists of people with mainly data science skills. They are responsible for researching on new algorithm, building POCs and train the model ONLY. Here, I see great potential in productivity increase and innovation. The second team would focus on generic development and deployment like building the application and pipeline for the ML model. This team would then also be responsible for bringing the product into production and fixing (non-ML-related) issues.
My 7 key takeaways to successfully building up an AI project that can be embedded in your organization (not only in banking):
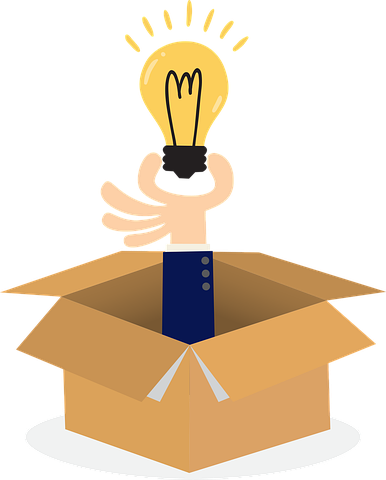
- Don’t agree on a project without ever having seen data.
- Before the project is kicked off, prepare and select data carefully – this is the basis for training a good model.
- Do not underestimate the labelling part. Solve annotation issues first, before thinking about modelling.
- Create POCs and small increments to validate the feasibility – it has many advantages. If it fails, at least you know early.
- POCs are valuable but should not get stuck at this stage. Try to build up an AI platform iteratively while bringing POCs into production.
- Have a decentralized team where the focus is only on applying new algorithms and building POCs.
- Have an operating/ supporting model in place where production is not maintained by the AI team.