If you don’t already know what the title is alluding to, let me give you a little hint:
What does even the world’s best tool for business intelligence and analytics (BIA) require?
∗ ∗ ∗ ∗ ∗ ∗ ∗ ∗ ∗ ∗
Are you ready to find out?
⇒ Half the answer lies in the following quote:
«You can have data without information, but you cannot have information without data.»
Daniel Keys Moran
And here is the full solution:
⇒ Congratulations are in order if you predicted high-quality data from the get-go!
∗ ∗ ∗ ∗ ∗ ∗ ∗ ∗ ∗ ∗
The α and Ω of BIA data
- Why is it crucial to achieve and maintain high data quality,
- What constitutes ‘high-quality’ data, and
- How to attain this imperative standard?
To help you out, I have compiled the most pertinent points into four nutshells below, based on my key take-aways from BIA lectures as well as other sources on the topic of data quality and management:
i. BIA(data) = decisions
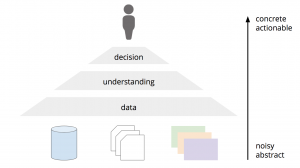
«The goal is to transform data into information and information into insight.»
Carly Fiorina
High-quality data is undoubtedly the most essential prerequisite for any meaningful attempt at taking advantage of business intelligence and analytics. It is thus of paramount importance for absolutely anyone and everyone who handles, uses or relies on data, i.e. no matter whether it be the data entry clerk or – even more so – the CEO / Board of Directors, to understand the necessity as well as, in consequence thereof, the commitment required for a solid base of trustworthy data!
ii. I(G) = O(G)
‘Garbage in, garbage out (GIGO)’ is a concept common to computer science as well as mathematics and implies that the input quality dictates the output quality. Although this expression is most frequently encountered in the context of software development, GIGO can also be used in other cases to refer to inevitably faulty decision-making as a result of poor data quality.
It should be noted that ‘garbage’ not only comprises inaccurate and incomplete data, but also irrelevant data. Pre-/Caution must therefore be exercised to ensure high-quality input data, the definition of which leads us straight to the next point.
iii. max(data quality) = ∑max(optimised attributes)
This might come as a surprise: There is actually no universal definition of data quality! Though on second thought, it makes sense that there is no absolute benchmark because data quality always depends on the context, namely the data’s intended use. Nonetheless, and generally speaking, high-quality data are determined by optimising the data quality dimensions (e.g. completeness, uniqueness, consistency, validity, accuracy, and timeliness) of the data collected such that they are fit for purpose, i.e. meet the needs of the data consumer.
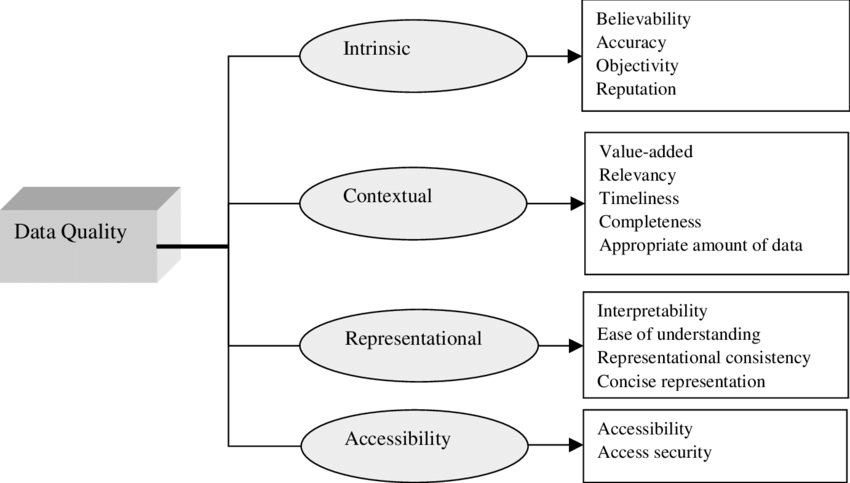
iv. BIA < think beyond (unbiased) data
Today, as more and more companies race towards digital, data-centric transformation, they should be acutely mindful of avoiding the pitfalls in relation to data-driven decision-making as well as (systemic / systematic) biases in data analytics and machine learning / artificial intelligence. In other words, resist data snooping and embrace diversity!
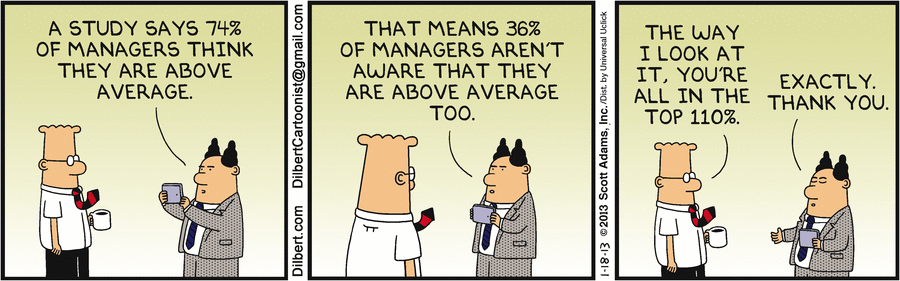
Whilst these four basics represent by no means an exhaustive list of what there is to know about the fundamentals of BIA, they hopefully spurred awareness of and reflection on some critical aspects. Lastly, my advice to all of you whom BIA may concern: Do spread the word (n.b. icons provided below for 7 easy ways) on the cornerstone of BIA necessitating QIQO – Quality In, Quality Out and, equally important, be sure to walk the talk so as to maximise your chances of data-informed SUCCESS!
Susan Liu is a project specialist, business analyst and divisional data manager at the Swiss Financial Market Supervisory Authority FINMA and blogs from the CAS Business Intelligence & Analytics class.